BETTER predictive power. FASTER scalable compute. DEEPER targets. CLEANER hydrocarbons.
X-Wave components for fully FWI based Model Building and Imaging
1. AWI Adaptive Waveform Inversion Avoids cycle skipping
2. RWI Reflection Waveform Inversion Extracts information from deepest reflection points
3. CWI Constrained Waveform Inversion Deals with highly-contrasting geology
4. QPSO Quantum Particle Swarm Optimisation Multi-parameter models
5. RIPSSCI Robust Inter Process Simple Socket Communication Interface Scalable fault-tolerant parallelisation
6. MJS Multi Job Submission dashboard Real-time monitoring
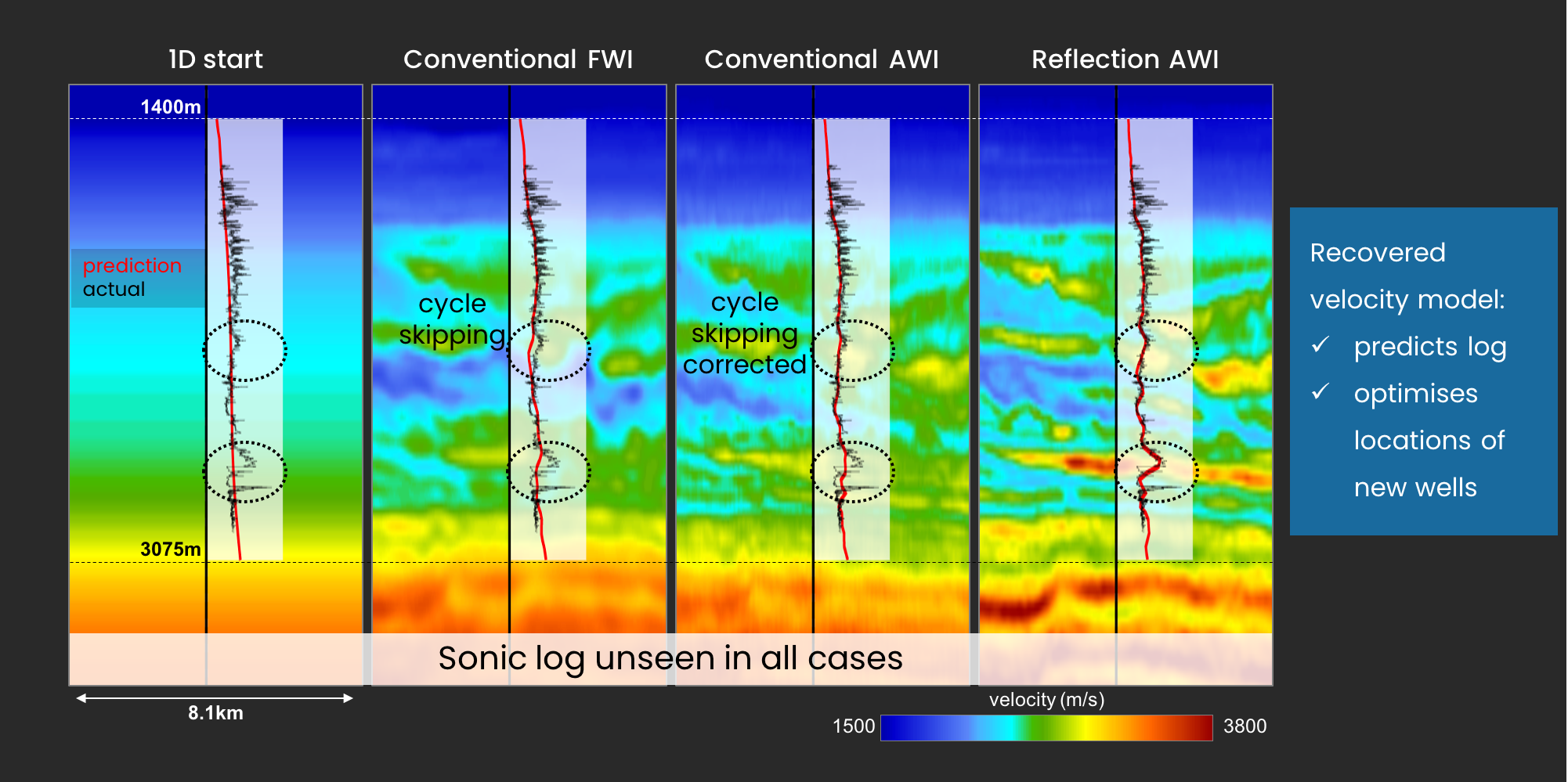
Full Set of Capabilities for Raw Data to Final Model in One Algorithm
Majority of the computational workload inside Full Waveform Inversion is performed by a finite-difference based wavefield simulation engine. This computes the forward and backpropagated wavefields needed for repeated simulation and cost function minimisation. This leverages the latest ARM-based c6g Graviton2 processors for which S-Cube were a selected launch partner. This enhanced FWI engine, with advanced capabilities such as structural-smoothing, is the basis of XWITM.
XWITM is designed to scale to thousands of square kilometres and parallelise over hundreds of thousands of shots without computational constraint. This is made possible through a communication protocol which is robust against interruptions.
S-Cube's novel MPI-free TCP-based-protocol - Robust Inter-Process Simple Socket Communications Interface (RIPSSCI) - was implemented to be part of the XWITM software used to perform the Woodside-AWS 1m core demo.
When running on the cloud, a dynamic pool of interruptible EC2 Spot instance types is provisioned per individual job with GPU's on demand for acceleration of total-variation based gradient conditioning.
To surpass the capability of conventional FWI, XWITM uses AWI and RWI cost functions.
Local inversion can be nested within a derivative-free QPSO outer search loop for navigating the misfit landscape, suppressing cross-talk using semi-global multi-parameter inversion (anisotropy, attenuation, elasticity). Multiple Spot Fleets are run simultaneously and as a result, a job which would take several weeks with traditional HPC on a typical on-premises system, can be completed in days on the cloud.
An API-based multi-job submission (MJS) system has been created to support hyperparameter tuning. This is able to fix key unknowns without having to guess values and run blind.
Cloud-Native High Performance Computing
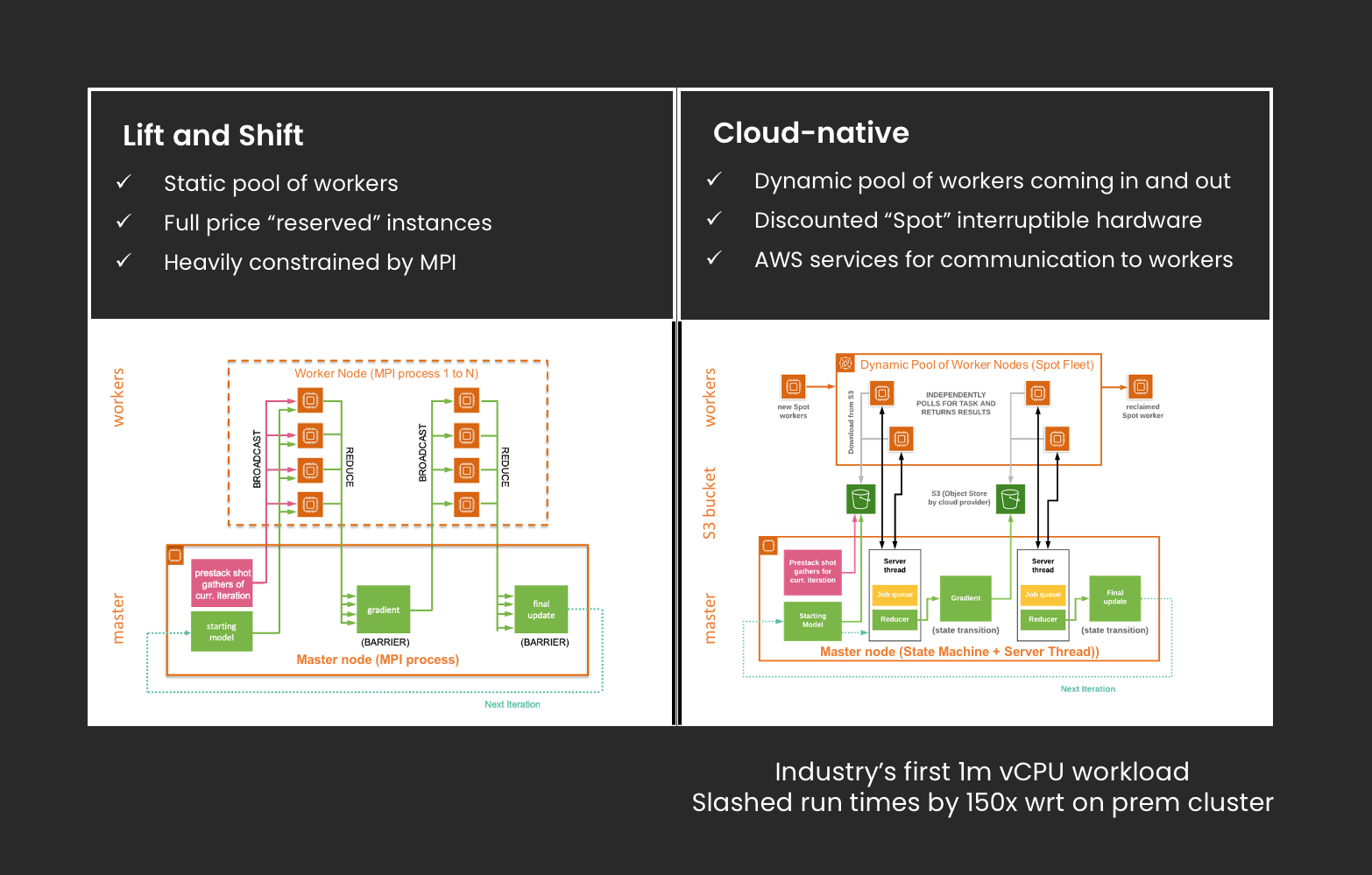
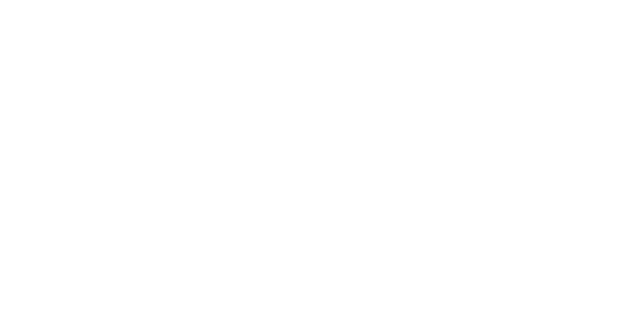
Run Full Waveform Inversion on the Cloud
Use S-Cube X-Wave FWI on AWS or Azure to redefine your salt geometry in under 4 weeks