Our mission:
leveraging our unparalleled expertise in Full Waveform Inversion, advanced seismic processing and depth imaging to optimize high-stakes drilling decisions
Who we are:
S-Cube Technologies is a forward-thinking, research-driven company, redefining seismic imaging by overcoming the conventional limitations. Founded on the belief that conventional FWI is progressing too slowly, we have identified a clear gap in the market applying fully FWI-based seismic imaging to both marine and land datasets for all subsurface energy targets.
Our offering:
S-Cube Technologies offers a full suite of PSTM, PSDM and FWI capabilities, for both land & marine, 2D & 3D data.
- Multi Cost Function XWITM (AWI-RWI-FWI) inversion sequence
- FWI Derived Velocity, Reflectivity & AVA volumes
- Kirchhoff Pre-Stack Time Migration
- Kirchhoff Pre-Stack Depth Migration
- Reverse Time Migration
- Full Azimuth Processing
- Multi-Dimensional Interpolation and Regularization – 2D/3D/4D/5D
- Noise and Multiple Attenuation
- Fault and Horizon Constrained Tomography
Land: US Gulf Coast
Tomography + XWI + RTM
Tomography | 20Hz XWI
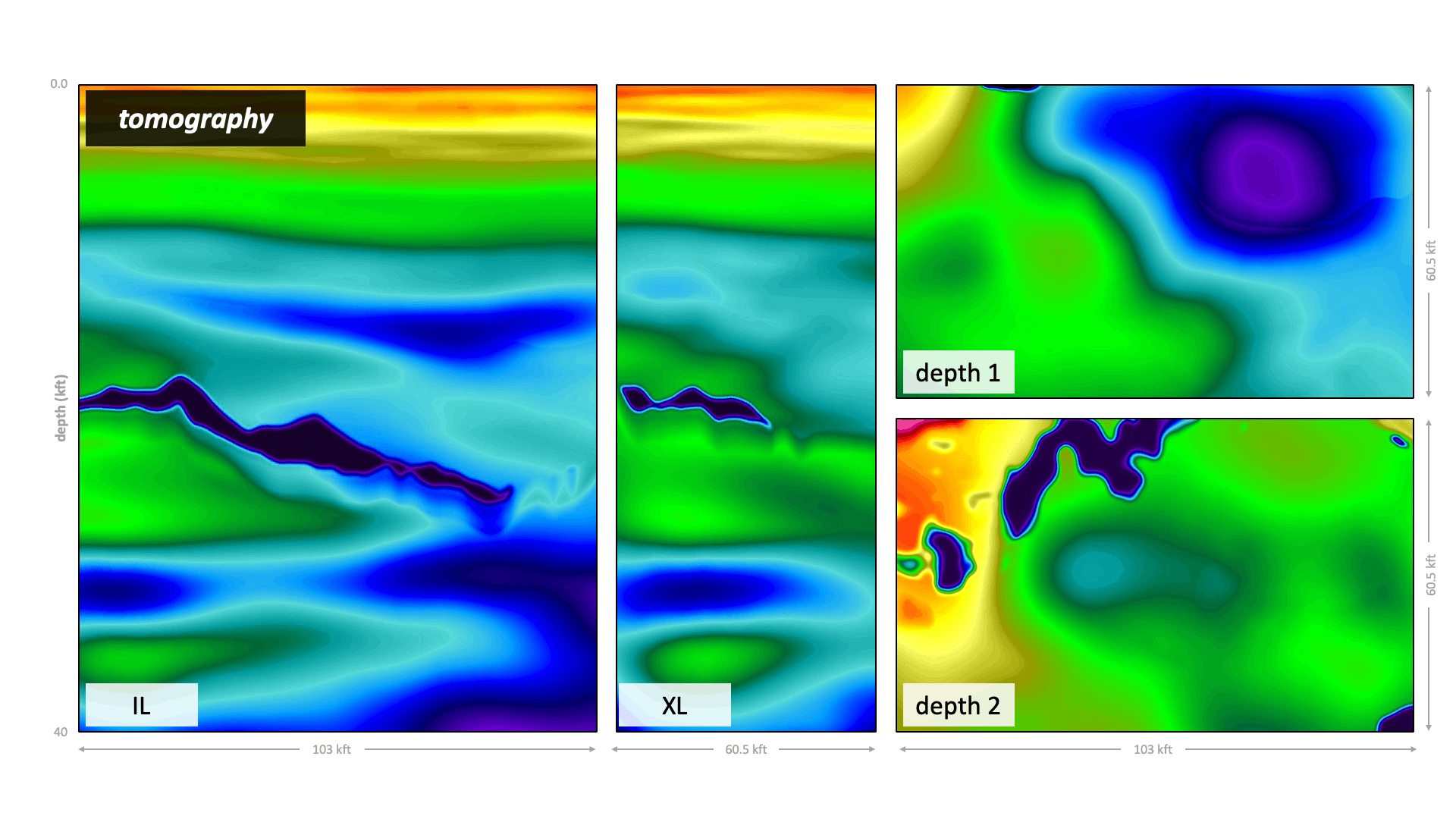
Legacy PSDM | 45Hz RTM with 20Hz XWI
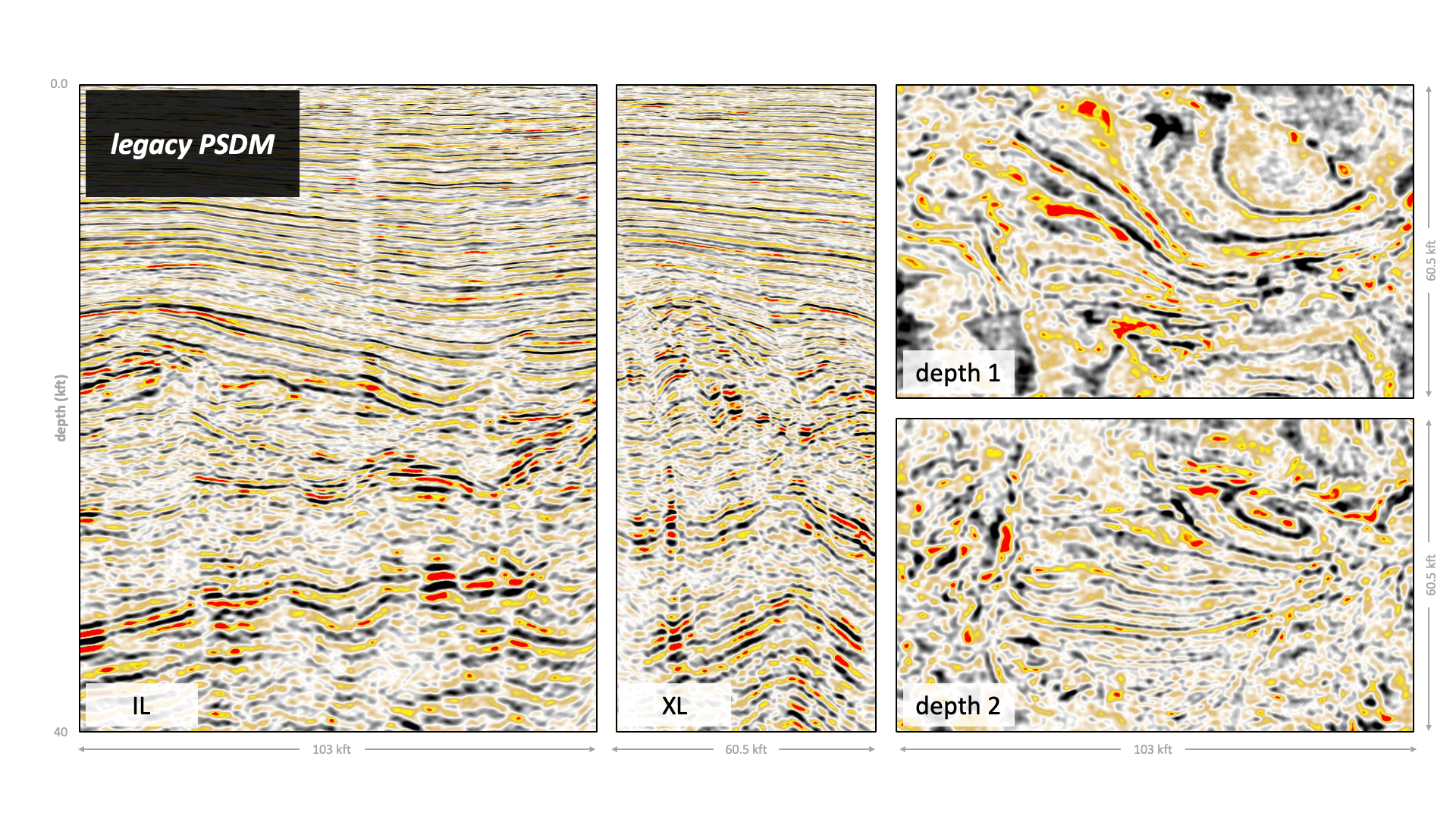
Field data | 20Hz XWI data
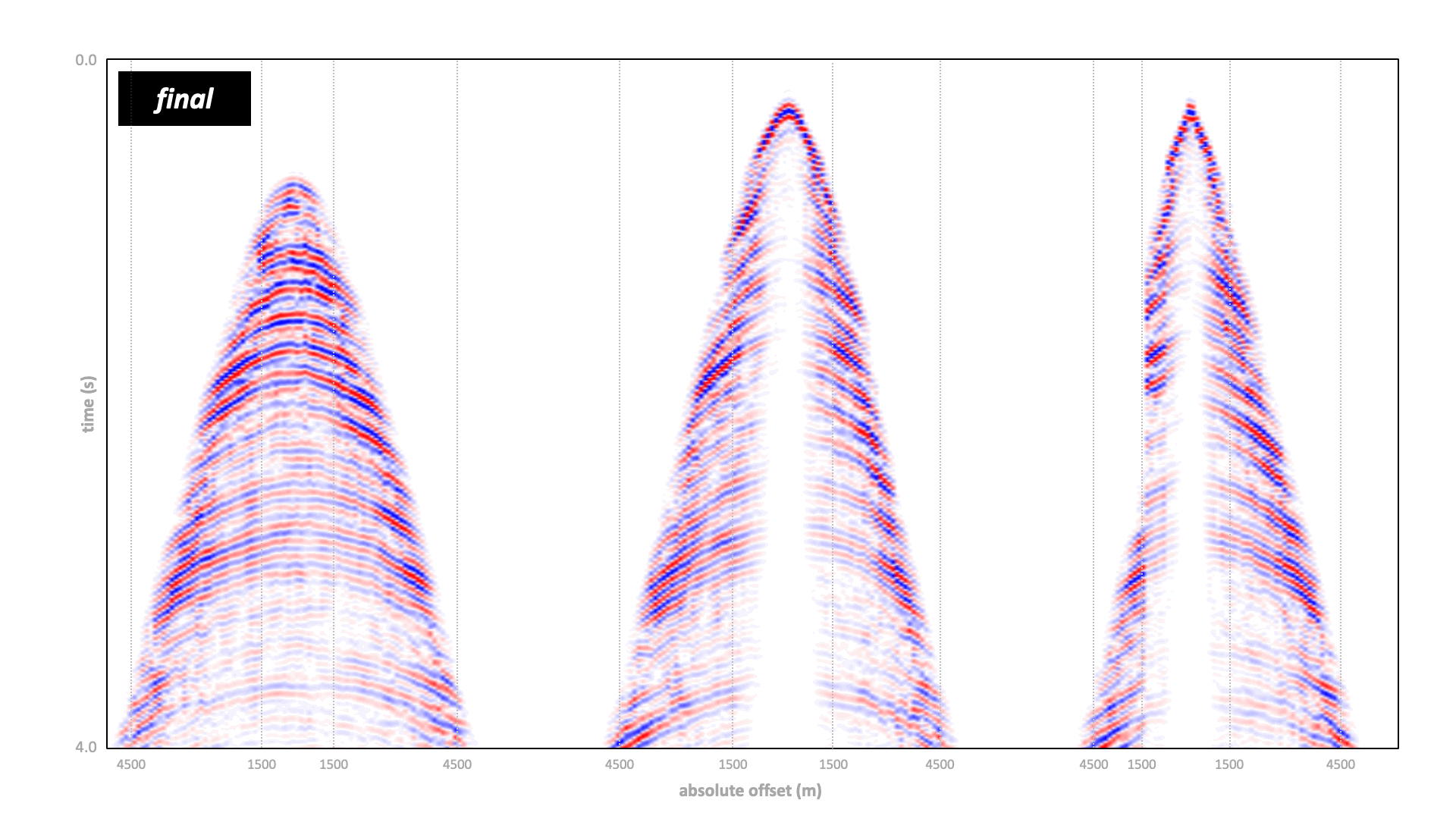
Marine: Brazil Santos Basin
XWI + RTM
Models and Reflectivity
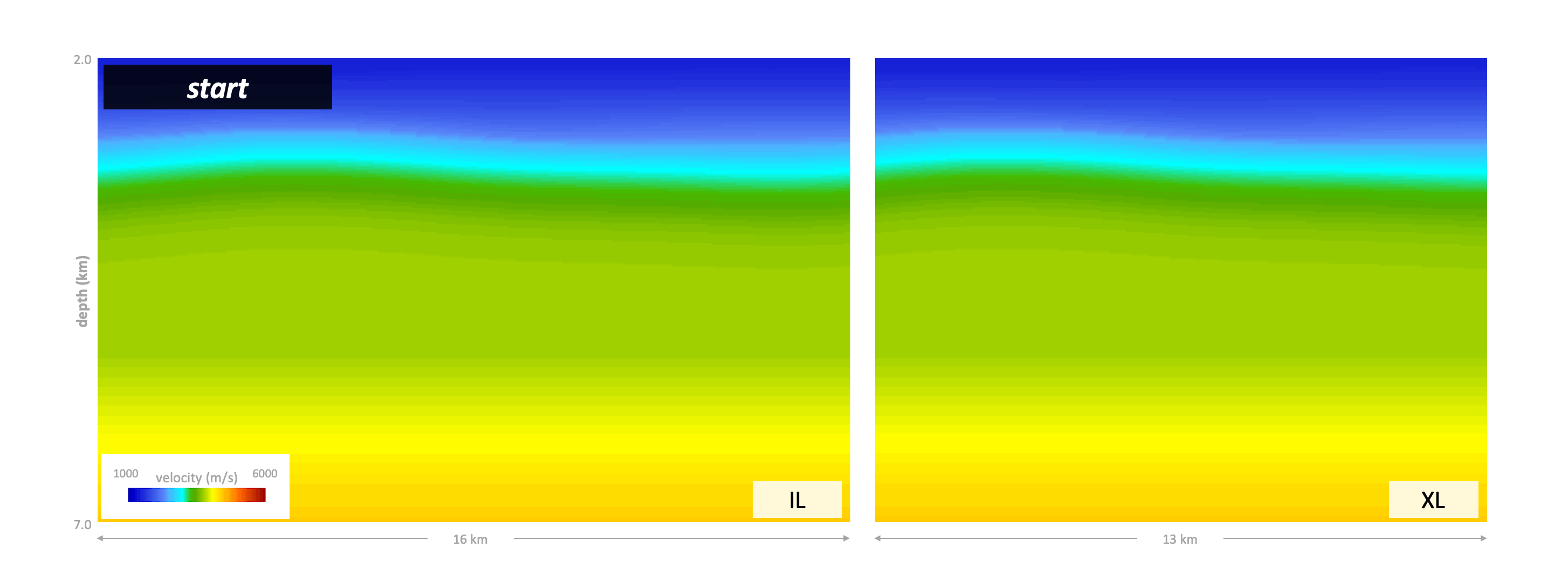
starts from no assumed salt
constrained AWI approximates the salt location
7Hz AWI-RWI determines the salt geometry and pre-salt trend
20Hz elastic AWI-RWI-FWI sharpens the definition
45Hz RTM
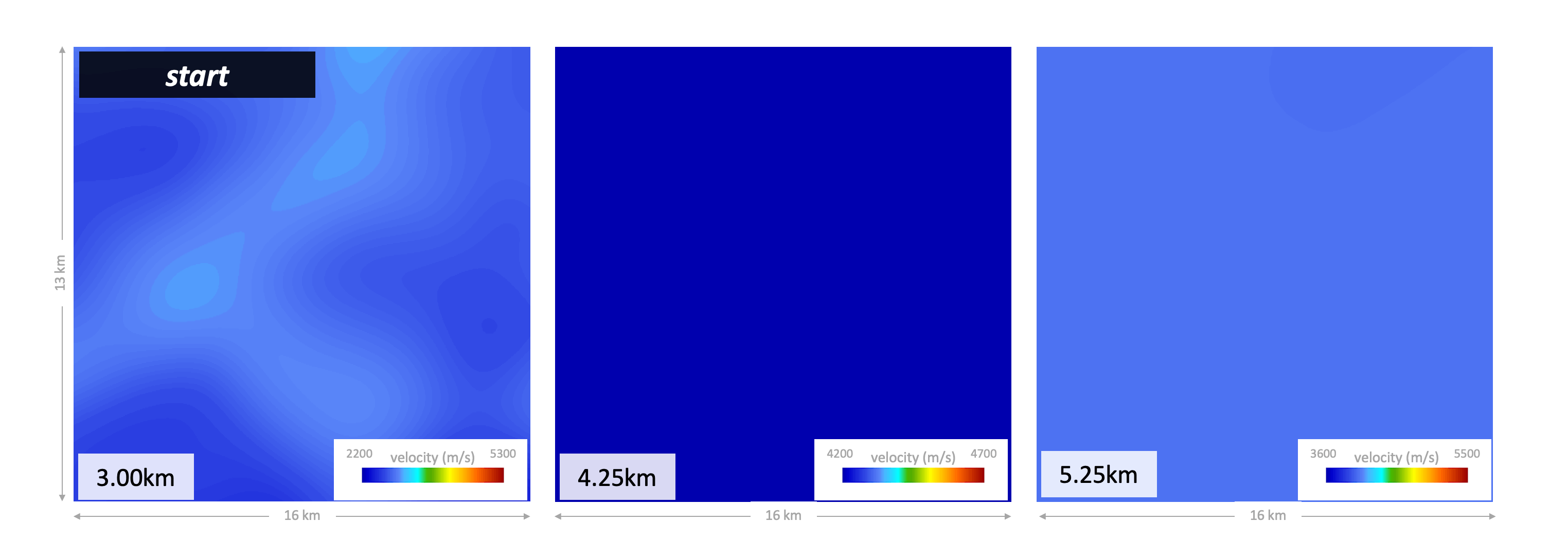
Data fit: start acoustic | final elastic | raw field
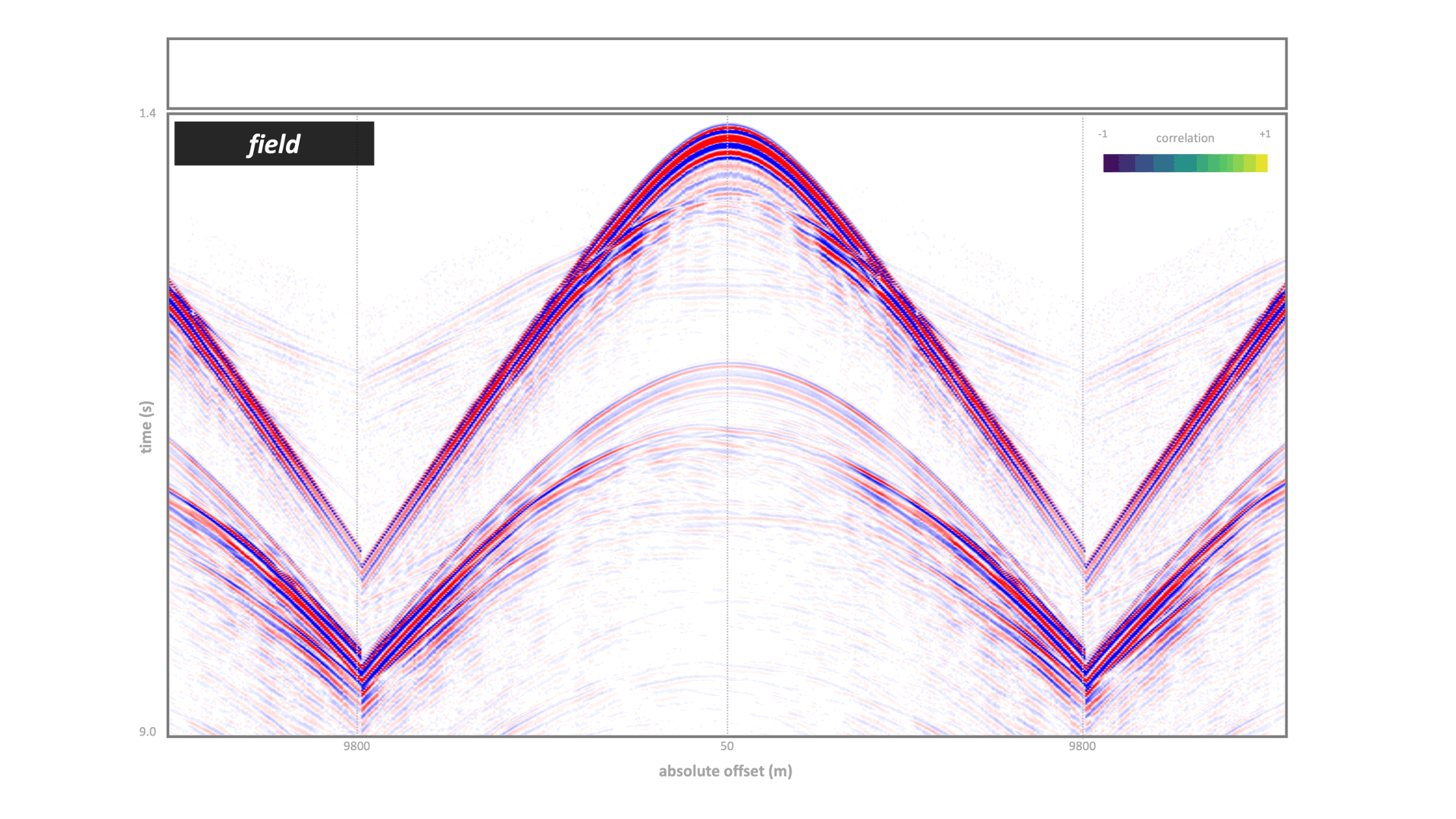